Data Analytics vs. Data Analysis
The meaning of the term analytics may seem indistinguishable from analysis, but these terms describe two distinct professional roles. This article aims to help you distinguish the business process of analytics from the data development practice of analysis.
Broadly speaking, data analytics refers to the development practices of multiple data pipelines, consuming raw data from its creation and organizing it at every stage, cleaning it, generating reports and visualizations, and eventually delivering these data products to the relevant consumers.
On the other hand, data analysis is a more concentrated activity that comprises one step in the wider data analytics pipeline. It aims to characterize data, producing predictions to give companies an on-the-go view of their data.
In order to design and produce competitive data systems, both data analysis and data analytics are important. Understanding their nuances is vital both for managers to make good hiring decisions and for data workers to develop strong career skills and maximize their value.
What is data analytics?
Data analytics is a broad concept, covering a variety of tools and processes. Specific roles like business intelligence, financial modeling, and marketing research all fall under the purview of data analytics. Even data analysis itself is a component of most data analytics systems. Professionals who work on the logistics and infrastructure components of data analytics are data engineers, whereas those who focus on the analysis components are data analysts.
Many people find it helpful to think of data analytics as a lifecycle of data development. Data analytics lifecycles provide outlines for each step of data analytics, from raw data ingestion, preparation, and modeling, to the data analysis itself. Every business has its own unique data ecosystem, and much of the work of data analytics is concerned with standardizing and optimizing the components in that ecosystem.
This means data analytics as a role requires a strong understanding of many different systems and the unique data they collect. Data analytics professionals have a grasp of processes from data storage to modeling and are capable of designing and implementing decisions made throughout the data analytics lifecycle.
Data analytics teams are also important because, when done well, analytics standardizes data processes and makes them accessible throughout the company. Communication, permissioning, and documentation are all also vital roles in data analytics.
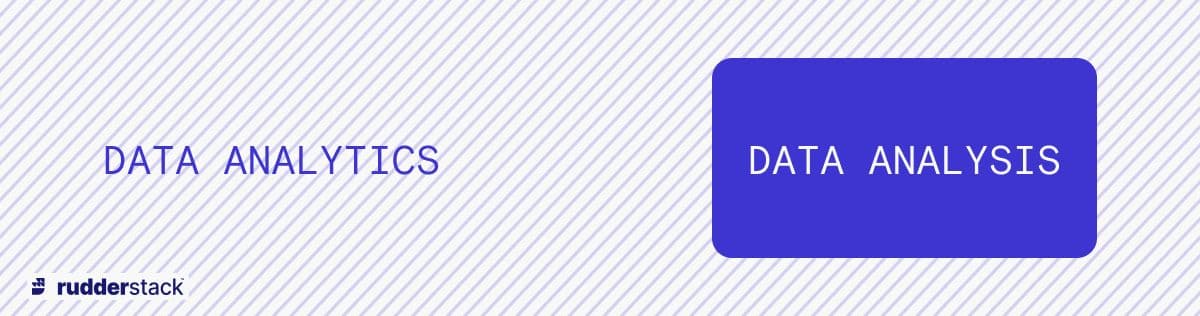
What is data analysis?
Data analysts have a comparatively limited (but no less valuable) role in data-driven companies. As the velocity of data is constantly increasing, it becomes more difficult to understand what information is available to decision-makers. This is where the data analysis comes in. Communicating insights and enabling data visibility to stakeholders throughout the company falls under the data analysis function.
Typically, data analysis fits into later stages of the data analytics lifecycle. Once data has been cleaned, or even been ingested and reinterpreted by models, a data analyst examines it and seeks to draw out interesting patterns.
These patterns are broken up into several characteristic directions of inquiry. The different approaches are important to distinguish because each requires domain and business-specific knowledge, so different analysts will have different aptitudes for each.
- Descriptive analysis – Descriptive analysis is used to answer factual questions about historical data. The aim of descriptive analysis is to give context and information to decision-makers about the state of a given set of metrics. Descriptive analysis can be the starting point for diagnostic analysis — further inquiry into causes of trends, research on correlated patterns, or other more in-depth reflection.
- Diagnostic analysis – It is useful to recognize what is happening, but it is also important to know why things happen, so that good trends can be amplified and bad ones short-circuited. Diagnostic analysis follows from descriptive analysis and often requires statistical expertise to determine the cause of a pattern.
- Predictive analysis – Predictive analysis uses modeling to suggest what is likely to happen based on existing trends. This is a more advanced form of data analysis. Cutting-edge predictive analysis often requires a good knowledge of statistics and modeling to achieve accurate results.
- Prescriptive analysis – Prescriptive analysis brings together other forms of analysis to determine how new events will affect observed data and thus recommend a course of action.
There is significant variability in the skills required for these data analysis techniques. Depending on the type of analysis being performed, one might need a background in statistics, an intuition for modeling, lighting-quick SQL skills, or an eye for visualization.
One commonality between any subtype of analysis is the requirement for a robust understanding of business problems and processes, so that important information can be separated from the chaff.
Differences between data analytics and data analysis
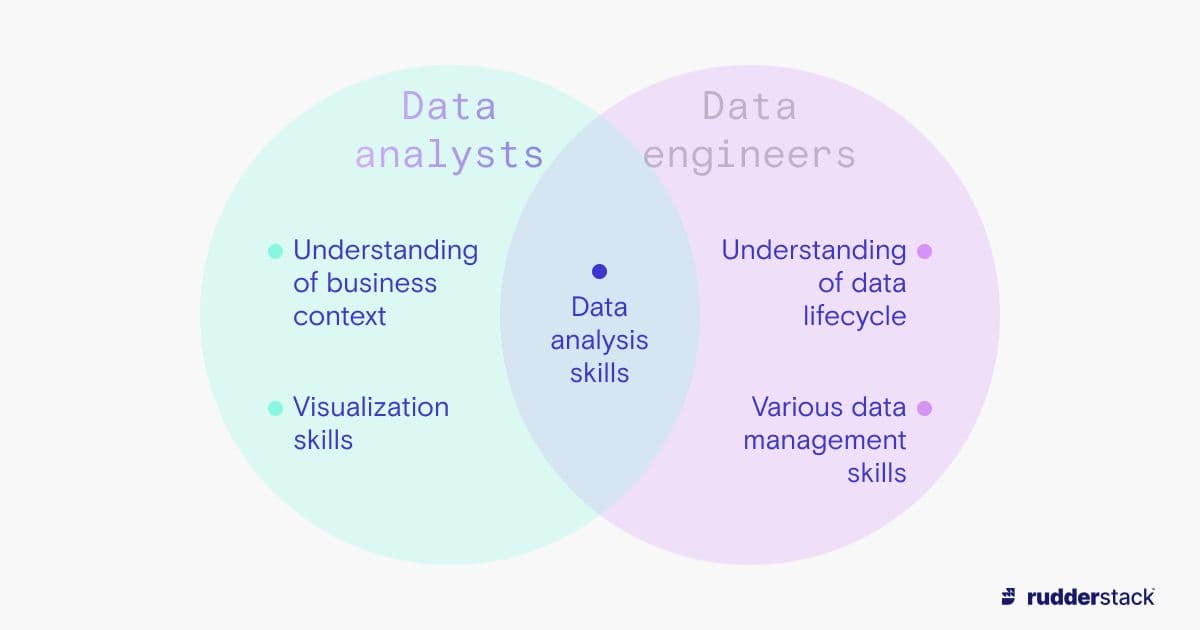
All data analysis is a component of data analytics, but not all the processes in analytics are analysis.
With that in mind, we will break down a few specific axes on which analytics departs from analysis.
General vs. specialized insights
Data analytics is a comprehensive process for managing the data flowing throughout a company. The analytics process must be adapted to all the various sources, formats, storage methods, stakeholders, and other specific requirements of data systems that arise during the organic growth of a company. Data analytics is tasked with solving that problem in the general case, with consideration of all the possible internal effects. This means that data analytics attempts to collect and prepare data for consumption by multiple end users from different areas such as business intelligence, marketing, sales, and, of course, data analysis.
Conversely, data analysis approaches problems in a vacuum. A data analyst processes and develops a dataset to examine a trend, but their processing for that dataset doesn’t need to be generalized to all company contexts. As such, data analysis often uses a subset of the additional information and metadata provided by data analytics. Analysis delivers a rapid-fire, comprehensible summary of available data.
Continuous vs. intermittent delivery
Data analytics primarily involves data summarization, trend finding, and prediction — similar-sounding but distinct from the goals of data analysis. Though data analytics teams develop observational products, these are usually pipelines that produce continuous knowledge and insight. For example, this would include regular ingestion and reporting on sales numbers.
Data analysis, on the other hand, is focused on drawing out specific information for a given moment — usually when a certain insight is requested. For example, a manager may request a snapshot of the current most popular product during an unexpected sales boom — something that is not part of the regular reports but is available on request. An analyst has less burdensome requirements in terms of developing infrastructure, and so can interpret events as they happen.
Analytics and analysis can be utilized together in many ways, a common use case being business intelligence dashboards: a collection of interesting trends developed by data analysts and formalized by data engineers.
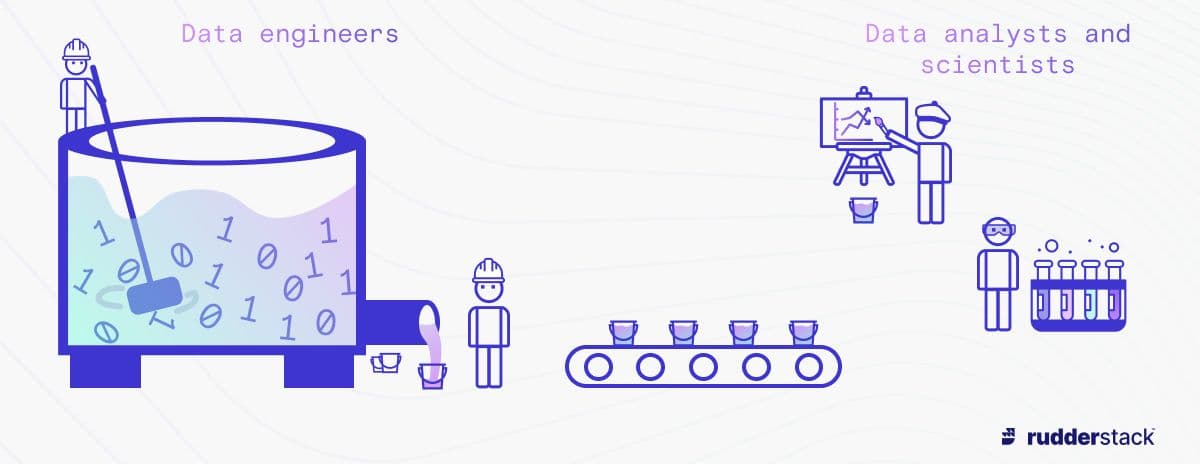
Data analytics, data analysis, and customer data platforms
Data-centric business requires both analysis tools and analytics lifecycles to function. In this article, we have discussed the purview of each process and the skills required for each role. While this is the core knowledge for distinguishing the terms, it is also worth examining the tools that you use to achieve the data-driven outcomes that will sustain and grow your business.
Picking the correct data systems is vital to having well-functioning analytics. It is well established that tools like data warehouses, data lakes, and big data systems are the norm for a world of vast and complex data. But just as data labor has become more specialized, so have the tools used to manage data.
Customer data platforms (CDPs) allow you to consume data from multiple sources for live analytics, and to store data for future analysis. A CDP is adapted specifically to optimize the typical use cases of data engineers who are working to extract value in a data analytics pipeline.
Fulfilling requirements such as personal information protection, identity resolution, and synchronization with third-party data vendors can be difficult and time-consuming for your engineers, creating drag on a data analytics lifecycle. By choosing the right CDP, you can avoid those headaches and ensure that your data process is streamlined, cost-effective, and compliant.
The Data Maturity Guide
Learn how to build on your existing tools and take the next step on your journey.
Build a data pipeline in less than 5 minutes
Create an accountSee RudderStack in action
Get a personalized demoCollaborate with our community of data engineers
Join Slack Community