Why Take a Warehouse-First Approach to Analytics

To take full advantage of analytics, it is essential to have an approach that allows you to effectively parse valuable insights from as wide an angle as possible, leveraging as much of your data as is available. According to a study by Gartner, poor data quality was one of the top three reasons organizations could not use analytics to make informed decisions. A warehouse-first approach to analytics addresses one of the root causes of poor data quality — siloed data in third-party tools — by creating a single source of relatable data.
The idea behind a warehouse-first stack is that you use your own data warehouse as a central “foundational” source of truth for your data modeling and, consequently, your organization. In doing so, you’ll be able to manage data storage directly and have complete visibility into each third-party data source and its relationship to other sources within your organization. This offers several advantages that result in an improvement in data quality, cost, and security.
For example, this means that you can aggregate different types of analytics data — clickstream, transactional, product, or identity sourced from tools like Mixpanel, Salesforce, Amplitude, or Clearbit — into one location and be able to clean, combine, and use that data however you want.
Here, we’ll break down the specific ways that the warehouse-first approach to analytics benefits not just the data team, but compliance and finance as well.
Aggregation of data sources leads to higher-quality analytics
When a company collects data, it will often use different extraction tools and applications depending on the data source, many of which have their own downstream data repositories, schema structures, and formatting. But to create meaningful conclusions, you need to be able to easily access and merge as much relevant information as possible since this will give a fuller picture of the customer journey.
For example, a company may use Amplitude to track user behavior within their product while also using Google Analytics to analyze web traffic. But when the need arises to draw combined insights from both data sources, it can be difficult as the tools cannot talk to each other directly because each dataset is siloed.
To solve this problem, a warehouse-first approach using a customer data platform (CDP) like RudderStack will collect data from both analytics tools and store them in a single modern data warehouse, allowing for easy access to multiple data sources. This leads to higher-quality analytics, as it becomes easier for cross-organizational teams to construct analytics that require data from multiple data sources.
Warehousing data reduces storage and latency costs
Maintaining data in multiple storage systems costs time and money.
You can reduce tooling costs by standardizing to a single platform. For example, consider a situation where you have multiple data sources that an analyst needs to use in order to generate a report. They will likely need to use a different tool for each data source as, frequently, there are different requirements for each system. This will require the company to pay for multiple tools compared to an approach where analysts can work directly from a single source.
Aside from reducing tooling costs, a warehouse-first approach also reduces the time cost associated with multiple sources. For example, if an analyst always has to pull data from multiple sources to generate analytics, it will take more time than pulling directly from a single source of data. By maintaining a single source of data, the time analysts need to spend gathering data is significantly reduced. Also, since only one source needs to be maintained for data quality, analysts get to work with a higher quality of data because error costs associated with pulling data from multiple sources are reduced.
Controlling storage allows for secure data
Collecting data has costs beyond the monetary outlay. When a company collects data, there are security, privacy, and compliance considerations for storing and accessing the data. For example, if you are working in the healthcare industry, one consideration for data storage is HIPAA compliance. Working with third-party storage systems dramatically increases the complexity and burden of compliance on your data engineers and compliance teams and radically increases the risk of exposure.
Storing your data in your own data warehouse allows you to implement your own data security protocols, such as encryption standards and the lifetime of data in the system. You are also able to control access to data in a more granular fashion through the warehouse-first approach. For example, you can employ data masking if you need to hide sensitive data, such as credit card numbers or personally identifiable information. Doing this allows you to generate reports that do not leak sensitive personal information.
In a solution that does not use a warehouse, storing masked versions of data might not be possible, meaning the process would need to be done manually, or the data can only be shared with privileged individuals.
In addition to controlling data storage, this approach also allows you to avoid sending data to third parties as you control the data storage. This allows you to worry less about the privacy and security of shared data and focus more on securing the data at the single source of truth. The result is that you control your security and privacy, allowing you to meet any compliance, security, and privacy requirements.
Building an analytics approach that works for you
Before you decide on an approach to your analytics stack, you need to consider the source, scope, and data requirements. Point solutions do provide utility for specific scenarios and use-cases, but are limited in their ability to provide a full view of customer engagement and the customer lifecycle. Warehouse-first approaches provide an excellent foundation for analytics and allow you to build complex and meaningful reports with ease. As a result, you can spend less time on low-level problems and focus on the crucial aspects of analytics.
Recent Posts
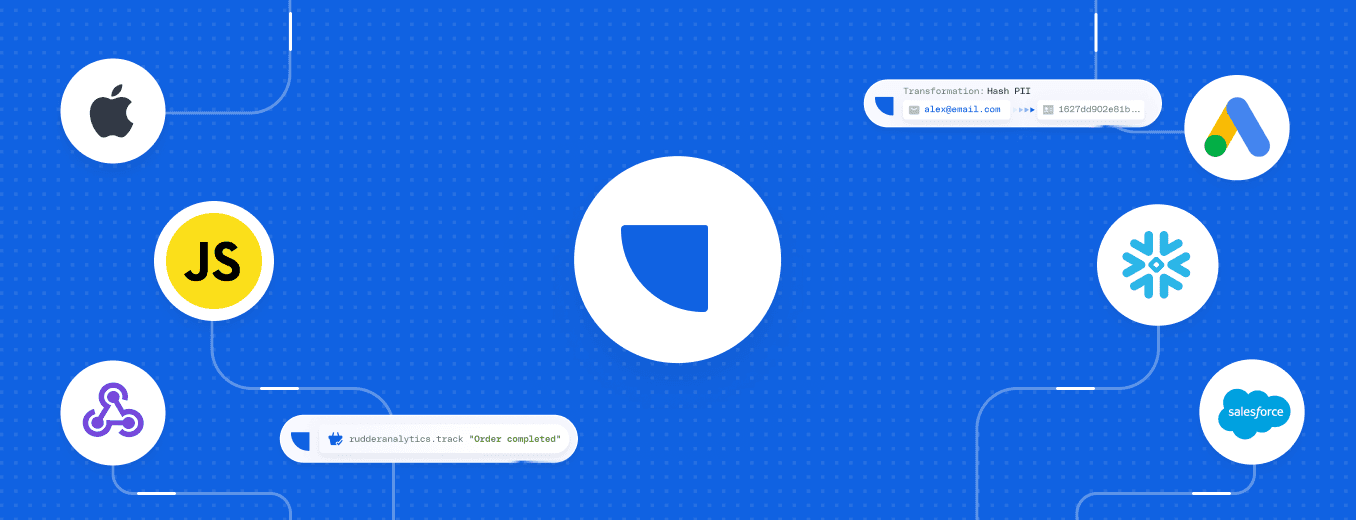
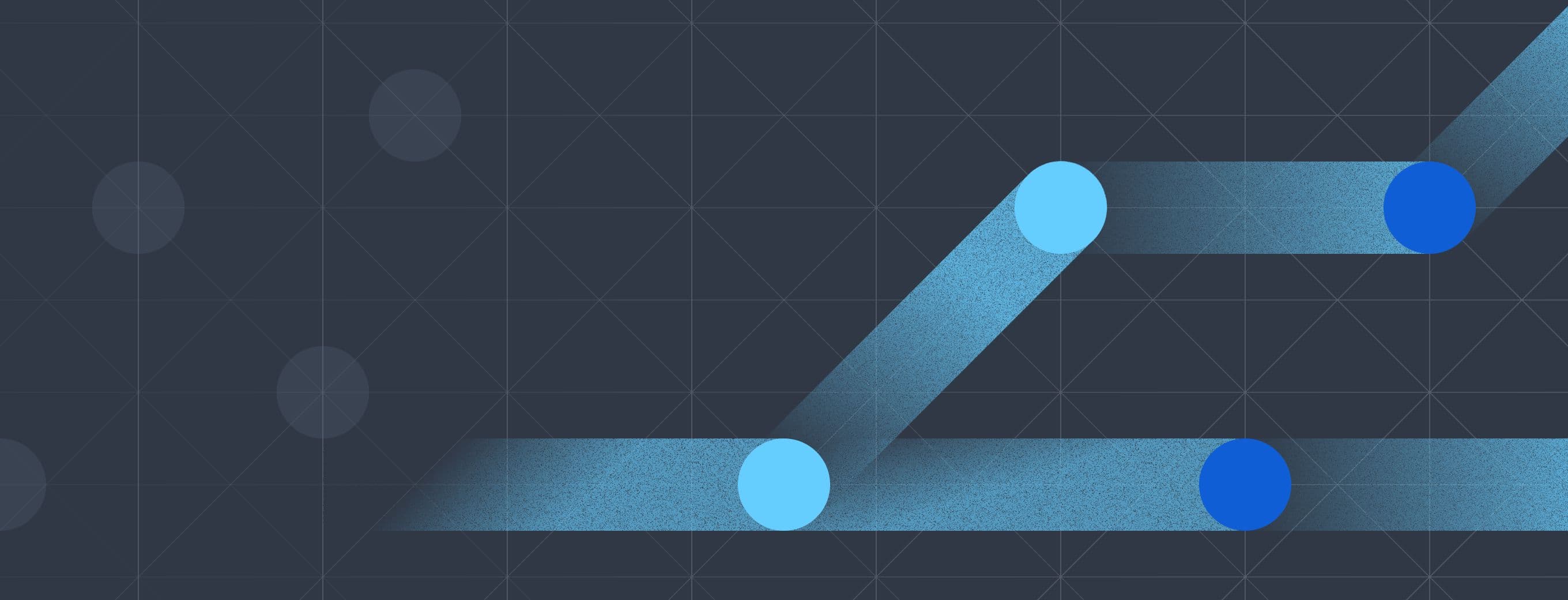
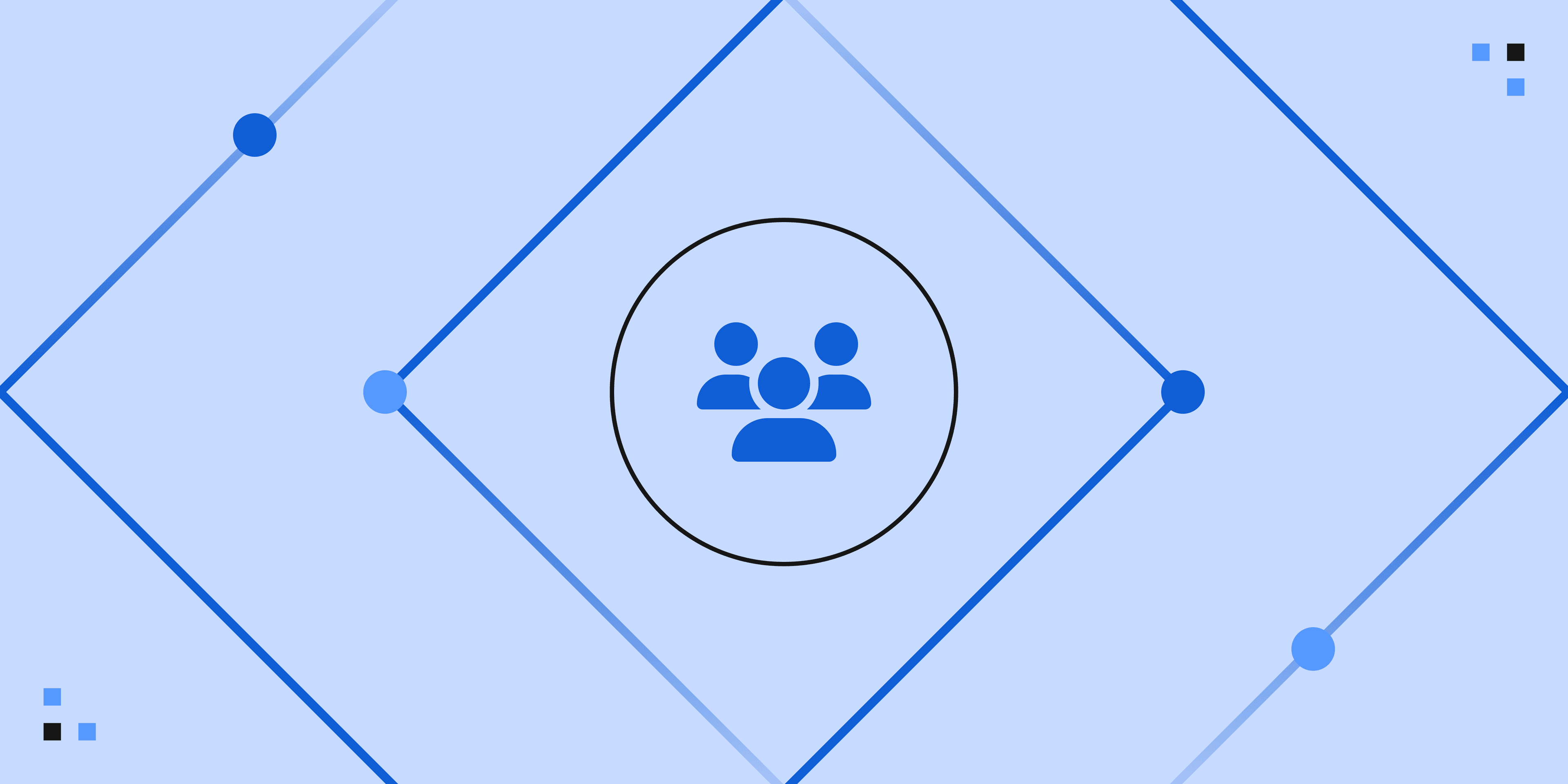